Using Learning Analytics to Understand and Support Collaborative Learning
Mohammed Saqr har undersökt hur social nätverksanalys kan användas för att förutsäga studenters framsteg och utmaningar i sina utbildningar och därmed främja samarbetet mellan studenter och mellan lärare och studenter i högre utbildning för att nå bättre resultat.
Mohammed Saqr
Professor Uno Fors, Stockholms universitet Docent Jalal Nouri, Stockholms universitet
Professor Barbara Wasson, University of Bergen
Stockholms universitet
2018-10-22
Using Learning Analytics to Understand and Support Collaborative Learning
Institutionen för data- och systemvetenskap
Using Learning Analytics to Understand and Support Collaborative Learning
Learning analytics (LA) is a rapidly evolving research discipline that uses insights generated from data analysis to support learners and optimize both the learning process and learning environment. LA is driven by the availability of massive data records regarding learners, the revolutionary development of big data methods, cheaper and faster hardware, and the successful implementation of analytics in other domains. The prime objective of this thesis is to investigate the potential of learning analytics in understanding learning patterns and learners’ behavior in collaborative learning environments with the premise of improving teaching and learning. More specifically, the research questions comprise: How can learning analytics and social network analysis (SNA) reliably predict students’ performance using contextual, theory-based indicators, and how can social network analysis be used to analyze online collaborative learning, guide a data-driven intervention, and evaluate it. The research methods followed a structured process of data collection, preparation, exploration, and analysis. Students’ data were collected from the online learning management system using custom plugins and database queries. Data from different sources were assembled and verified, and corrupted records were eliminated. Descriptive statistics and visualizations were performed to summarize the data, plot variables’ distributions, and detect interesting patterns. Exploratory statistical analysis was conducted to explore trends and potential predictors, and to guide the selection of analysis methods. Using insights from these steps, different statistical and machine learning methods were applied to analyze the data. The results indicate that a reasonable number of underachieving students could be predicted early using self-regulation, engagement, and collaborative learning indicators. Visualizing collaborative learning interactions using SNA offered an easy-to-interpret overview of the status of collaboration, and mapped the roles played by teachers and students. SNA-based monitoring helped improve collaborative learning through a data-driven intervention. The combination of SNA visualization and mathematical analysis of students’ position, connectedness, and role in collaboration was found to help predict students’ performance with reasonable accuracy. The early prediction of performance offers a clear opportunity for the implementation of effective remedial strategies and facilitates improvements in learning. Furthermore, using SNA to monitor and improve collaborative learning could contribute to better learning and teaching.
Relaterade länkar
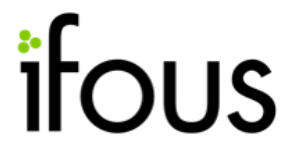
Undervisa i engelska med digitala verktyg
Kurs för dig som undervisar i engelska med digitala verktyg.
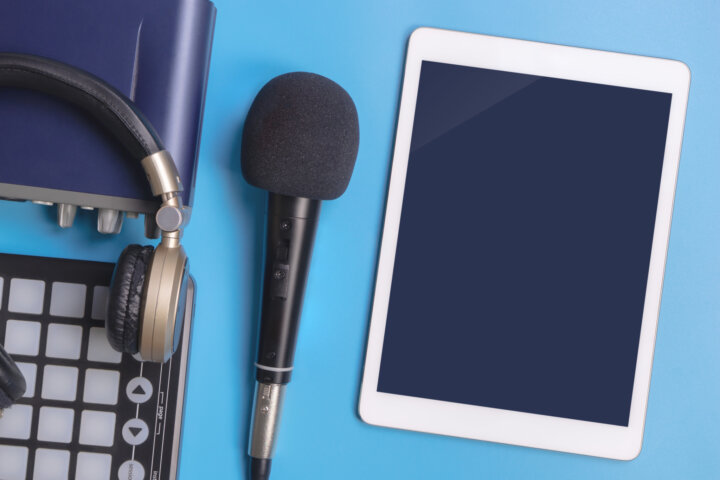
Köp den för 749 kr.
Kursintyg ingår.